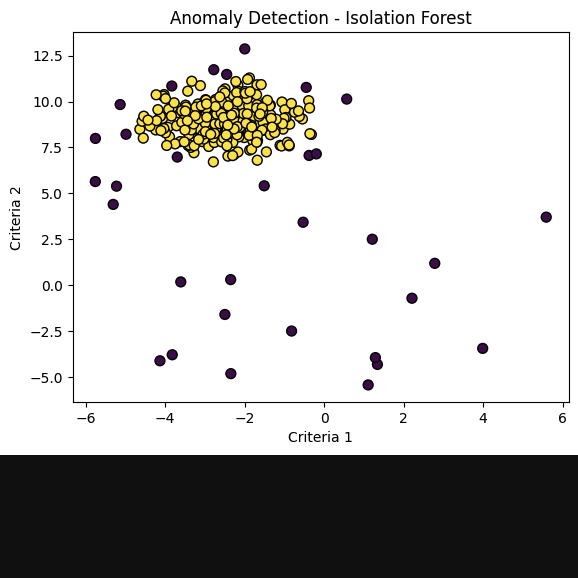
-
Project Title
Residual Biomass Maximization through Anomaly Detection in AgriTech
-
Timeline:
1.5 Months
-
Tech Stack:
Python
-
Demo Link:
-
Client Name:
Project Detail:
The project aims to leverage data-driven techniques to detect and rectify anomalies in agricultural processes. The objective is not only to identify these irregularities but also to optimize the process to maximize residual biomass, a crucial resource in sustainable agriculture.
Problem Statement:
With the growing emphasis on sustainable farming, maximizing residual biomass becomes paramount. However, undetected anomalies in agricultural processes can lead to wastage and reduced biomass yields.
Solution:
By harnessing machine learning algorithms, we've developed a system that continuously monitors various agricultural metrics and flags any irregular patterns. Once detected, the system suggests corrections to enhance efficiency and optimize for higher biomass residuals.
Features:
- Real-time Anomaly Detection: Continuous monitoring and instant alerting on irregularities.
- Predictive Analysis: Using past data to predict potential future anomalies.
- Optimization Recommendations: Suggesting process alterations to maximize biomass.
- Interactive Dashboards: Visual representations of agricultural metrics and anomalies.
Use Cases:
- Sustainable Farming: Ensuring minimal wastage and maximizing biomass for green energy projects.
- Quality Control: Maintaining a consistent quality of produce by minimizing anomalies.
- Efficiency in Farming Operations: Reducing costs related to wastage and sub-optimal processes.
Data Science Specific Points:
- Data Collection: Data was sourced from multiple farms using IoT devices capturing soil quality, weather conditions, crop health, and more.
- Data Analysis: Utilized deep learning models to discern patterns and detect anomalies within large datasets. Historical data was used to train the model to recognize typical patterns and thus detect deviations.
- Results: Achieved a substantial reduction in undetected anomalies, leading to an average increase of 18% in residual biomass across collaborating farms.
Technologies Used:
- Programming Languages: Python
- Frameworks: Custom Algorithms
- Database Systems: PostgreSQL, Kafka